Generative AI vs Predictive AI : A Comprehensive Exploration
AI is transforming industries and creating new job opportunities. This blog will delve into Generative AI vs Predictive AI, exploring their unique features and applications. We’ll examine what Generative AI vs Predictive AI are, how they function, their use cases, the challenges they encounter, job prospects, and future developments, based on the latest information and reports.
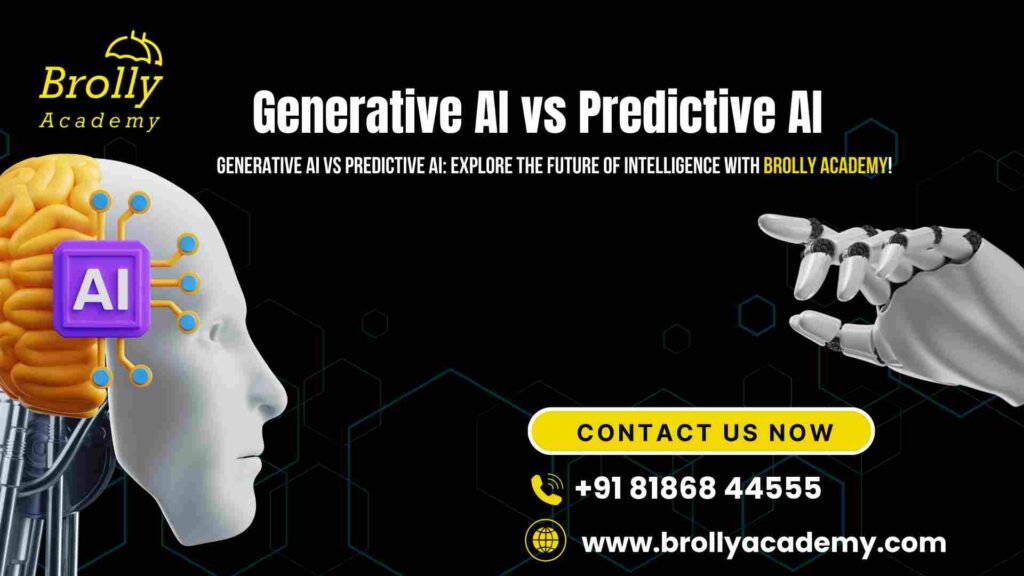
Introduction
The AI market is projected to reach $190.61 billion by 2025, with a compound annual growth rate (CAGR) of 36.62% from 2020 to 2025. This growth is driven by the increasing adoption of AI technologies across various sectors, including finance, healthcare, marketing, and creative industries. Notably, a report from McKinsey highlights that generative AI could contribute between $2.6 trillion and $4.4 trillion annually to the global economy, emphasizing its substantial impact. In the context of Generative AI vs Predictive AI, while generative AI focuses on creating new content and data, predictive AI analyzes historical data to forecast future trends. Understanding the differences between these two AI types is crucial for businesses looking to leverage their unique capabilities effectively. As the market continues to expand, the interplay between generative AI and predictive AI will shape innovation and drive economic growth.
Understanding Generative AI
• Definition and Mechanisms
Generative AI is a part of artificial intelligence that creates new data and content. Unlike regular AI, which mainly looks at and predicts from existing data, generative AI makes new outputs like text, images, and complex data forms. The main idea behind generative AI is to understand the patterns in the training data and create new examples that fit those patterns.
This usually happens in three steps:
Training: The model learns from a large dataset, finding patterns and connections in the data.
Customization (Fine-tuning): The model is adjusted to perform better on certain tasks or datasets.
Generation: The trained model creates new data that has similarities to the training data but is original and different.
• Key Technologies
Generative AI uses advanced machine learning methods, such as:
Generative Adversarial Networks (GANs): These involve two neural networks—a generator and a discriminator. The generator makes new data, while the discriminator checks it against real data and gives feedback to help the generator improve.
Variational Autoencoders (VAEs): VAEs create new data by learning a simplified version of the input data, which helps in producing new examples similar to the original data.
Large Language Models (LLMs): Models like OpenAI’s GPT series can create clear and relevant text based on prompts, demonstrating the capabilities of generative AI in understanding and producing language.
• Applications
Generative AI is useful in many areas:
Content Creation: Artists and writers utilize generative AI to produce unique artworks, music, and text, boosting creativity and artistic skills.
Data Augmentation: In machine learning, generative AI generates synthetic data to enhance model training, particularly when real data is limited.
Simulation and Synthetic Data Generation: Generative AI can produce synthetic data for simulations, allowing for testing and assessment in controlled settings.
Anomaly Detection: By understanding normal data patterns, generative AI models can spot anomalies or outliers, making them valuable for fraud detection and system oversight.
• Use Cases
Generative AI is being used more and more in different fields:
Entertainment: AI creates music and art for movies and video games, boosting creativity and cutting production costs.
Healthcare: Generative AI can mimic patient data for training healthcare models, protecting patient privacy and improving accuracy.
Marketing: Companies use generative AI to make personalized marketing materials, like social media posts and emails, that fit each customer’s likes.
Understanding Predictive AI
Definition and Mechanisms
Predictive AI is about looking at past data to guess what might happen in the future. It uses statistics and machine learning to find patterns and trends, helping businesses make smart choices based on what the data shows.
The steps in predictive modeling usually include:
Data Collection: This means gathering past data that relates to the issue, like sales numbers, customer actions, or market changes.
Model Training: Different methods, like regression analysis, decision trees, and neural networks, are used to teach the predictive models. These models learn to see connections in the data and make predictions based on what they have learned.
Prediction Phase: After training, the model can look at new data and give predictions, like estimating future sales or spotting possible risks.
Key Technologies
Predictive AI uses important technologies such as:
Regression Analysis: This is a statistical technique that helps understand how a dependent variable relates to one or more independent variables, which aids in making predictions from past data.
Decision Trees: These are diagram-like tools that show decisions and their potential outcomes, helping to clarify the decision-making process.
Time Series Analysis: This involves methods for examining data points collected over time to find trends, seasonal changes, and cycles, which helps in making precise forecasts.
Applications
Predictive AI is commonly applied in many fields, such as:
Finance: Banks and financial companies utilize predictive AI for evaluating risks, spotting fraud, and improving investment strategies.
Healthcare: Predictive models help identify patient risk factors, allowing for better healthcare management and resource distribution.
Retail: Stores use predictive AI to predict demand, manage inventory efficiently, and tailor marketing efforts to consumer habits.
Manufacturing: Predictive maintenance employs AI to anticipate equipment breakdowns, enabling manufacturers to plan maintenance in advance.
Use Cases
Predictive AI is widely used in various fields:
Customer Relationship Management (CRM): Companies apply predictive analytics to find potential customers and enhance interactions, leading to better sales and happier clients.
Supply Chain Management: Predictive AI helps predict supply chain issues, allowing businesses to manage inventory and logistics in advance.
Insurance: Insurance companies utilize predictive models to evaluate risk and set premium prices based on past data and customer information.
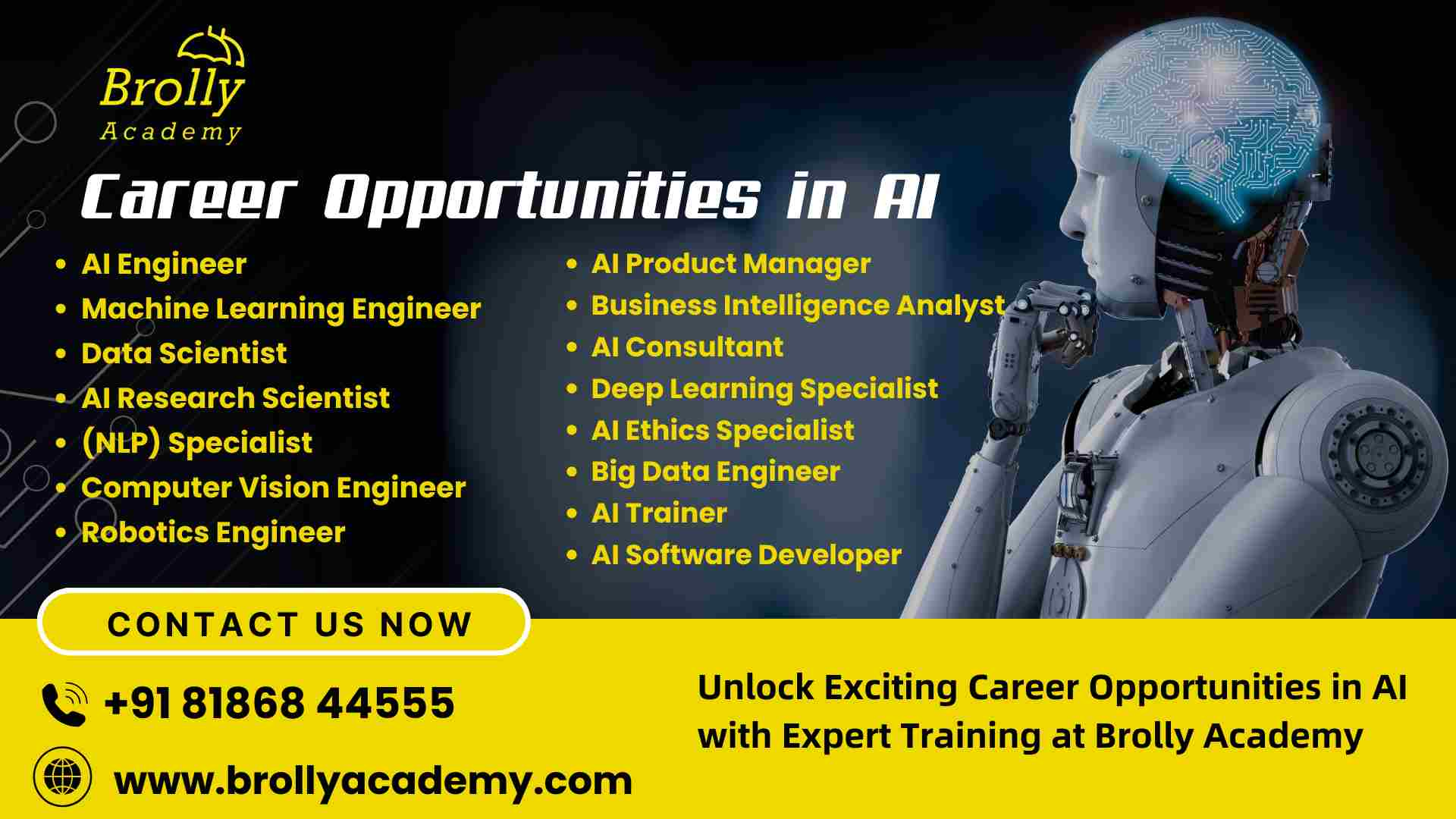
Career Opportunities in AI
The rise in demand for AI technologies is creating more job opportunities in generative and predictive AI. Let’s explore the different career options in these areas.
Jobs in Generative AI
Generative AI Engineer: This role involves creating and developing generative models. It requires knowledge of machine learning, deep learning tools, and programming languages like Python.
Machine Learning Researcher: This position focuses on improving generative AI through research and the creation of new algorithms and models.
AI Product Manager: This person manages the creation and launch of generative AI products, ensuring they fit market needs and align with the company’s goals.
AI Ethicist: This role ensures that generative AI technologies are developed and used responsibly, tackling issues like bias and transparency.
Data Scientist – Generative Models: This job uses generative AI methods to analyze data and gain insights, often collaborating with business teams to implement results.
AI Content Creator: This role involves using generative AI tools to create various types of content, such as writing, music, or art, often working with artists and designers.
Robotics Engineer – Generative AI: This position focuses on incorporating generative AI into robotic systems, improving their ability to learn and adapt to their surroundings.
Jobs in Predictive AI
Predictive Analyst: Studies data to find patterns and make predictions, commonly in fields like finance, healthcare, and marketing.
Data Scientist: Uses predictive modeling to gain insights from data, aiding organizations in making informed choices.
Business Intelligence Analyst: Works with predictive analytics to shape business strategies, improving operations and decision-making.
Risk Analyst: Focuses on evaluating and managing risks through predictive models, especially in finance and insurance.
Operations Research Analyst: Uses predictive analytics to boost efficiency and decision-making in organizations.
Machine Learning Engineer: Creates and applies predictive models, needing strong programming skills and knowledge of machine learning techniques
Key Differences Between Generative AI and Predictive AI
Generative AI vs Predictive AI
Parameters | Generative AI | Predictive AI |
---|---|---|
Objective | Generates new, original content or data | Predicts and analyzes existing patterns or outcomes |
Function | Creates new information or content | Makes predictions based on existing data |
Training Data | Requires diverse and comprehensive data | Requires historical data for learning and prediction |
Examples | Text generation, image synthesis | Forecasting, classification, regression |
Learning Process | Learns patterns and relationships in data | Learns from historical data to make predictions |
Use Cases | Creative tasks, content creation | Business analytics, financial forecasting |
Challenges | May lack specificity in output | Limited to existing patterns, may miss novel scenarios |
Training Complexity | Generally more complex and resource-intensive | Requires less complex training compared to generative models |
Creativity | Creative and produces things that have never existed before | Lacks the element of content creation |
The Symbiosis of Generative and Predictive AI
Generative AI vs Predictive AI serve distinct roles but can complement each other effectively. Generative AI can produce synthetic data to enhance predictive models, making them more accurate and reliable. Conversely, predictive AI can guide generative processes by identifying which types of content are likely to be effective or appealing.
Hybrid Models
The combination of Generative AI vs Predictive AI has led to hybrid models that integrate the strengths of both. These models create new content while also analyzing and forecasting trends, resulting in more advanced applications across various fields.
For example, a hybrid model might generate customized marketing content using predictive analytics to understand customer preferences and behaviors. This approach can enhance customer engagement and drive sales.
Future Trends in AI
As AI technology advances, the lines between generative and predictive AI may become less clear. Combining these methods could create more sophisticated systems that understand context, produce relevant content, and make precise predictions.
Improvements in Natural Language Processing (NLP) are key to the overlap of generative and predictive AI. Developments in NLP models, like OpenAI’s GPT series, show the capability to create coherent text while grasping context and intent. This progress can enhance chatbots, virtual assistants, and tools for content creation.
Market Trends and Data
• Market Insights: The global generative AI market was valued at $3.2 billion in 2022 and is expected to grow at a rate of 34.3% annually from 2023 to 2030, according to Fortune Business Insights. Meanwhile, the predictive analytics market is projected to reach $27.4 billion by 2026, with a growth rate of 22.0% from 2021.
• Adoption Trends: A Deloitte survey revealed that 64% of organizations are looking into or using generative AI technologies, while 53% are utilizing predictive analytics in their operations.
• Investment Patterns: Investment in generative AI startups has increased significantly, with over $1 billion invested in the first half of 2023. Predictive analytics also draws considerable investment, especially in healthcare and finance, where data insights are essential.
Ethical Considerations
The growth of generative and predictive AI brings up ethical concerns about data use, bias, and potential misuse. Organizations need to focus on ethical AI practices, ensuring their models are transparent, fair, and accountable.
• Bias Reduction: Both generative and predictive AI can reflect biases found in their training data. Organizations should adopt methods to identify and reduce these biases in their models.
•Data Privacy: As AI systems increasingly rely on personal data, ensuring data privacy and compliance with regulations (such as GDPR) is paramount.
• Transparency: Stakeholders should be able to understand how AI models make decisions. This transparency is crucial for building trust and accountability in AI systems.
•Regulatory Frameworks: As AI technologies evolve, regulatory frameworks will need to adapt to address the ethical implications and ensure responsible AI deployment.
Limitations and Potential Risks of AI Technologies
Artificial Intelligence (AI) has rapidly evolved, transforming various sectors by enhancing efficiency and automating tasks. However, despite its many advantages, AI technologies come with significant limitations and potential risks that must be carefully considered.
Key Limitations of AI
- Dependence on Data Quality
AI systems rely heavily on the data they are trained on. If the data is biased, incomplete, or unrepresentative, it can lead to inaccurate or discriminatory outcomes. This is particularly concerning in critical areas like healthcare and law enforcement, where flawed AI decisions can have serious consequences. - Lack of Emotional and Social Understanding
AI struggles to comprehend human emotions, social cues, and cultural nuances. This limitation can hinder its effectiveness in applications requiring empathy, such as mental health support or customer service interactions. AI’s inability to understand context can lead to misinterpretations and errors in communication. - Ethical and Privacy Concerns
The deployment of AI raises ethical questions regarding data privacy, informed consent, and algorithmic bias. These concerns can overshadow the benefits of AI, especially when technologies are implemented without adequate oversight. The potential for surveillance and loss of privacy is a significant risk associated with AI systems. - Complexity and Resource Intensity
Many advanced AI algorithms are resource-intensive, requiring substantial computing power and specialized hardware. This can make AI technologies inaccessible to smaller organizations and raises environmental concerns due to high energy consumption. - Limited General Intelligence
Current AI systems excel in narrow tasks but lack the ability to generalize knowledge across different domains. This limitation restricts their adaptability and effectiveness in dynamic environments where new information constantly emerges. - Bias and Discrimination
AI systems can perpetuate and amplify existing societal biases if trained on biased data. For example, facial recognition technologies have shown higher error rates for certain demographic groups, raising concerns about fairness and equity in AI applications.
Potential Risks of AI Technologies
- Job Displacement
The automation of tasks traditionally performed by humans can lead to significant job losses. While AI can enhance productivity, it may also result in economic inequality and social disruption as workers are displaced from their roles. - Lack of Transparency and Explainability
Many AI algorithms, particularly those based on deep learning, operate as “black boxes,” making it difficult to understand how decisions are made. This lack of transparency can undermine trust in AI systems and complicate accountability when errors occur. - Security Vulnerabilities
AI systems can be susceptible to adversarial attacks, where malicious actors manipulate input data to deceive the AI. This vulnerability poses risks in critical applications, such as autonomous vehicles or security systems, where incorrect decisions can have dire consequences. - Overconfidence in AI Systems
AI systems may exhibit excessive confidence in their outputs, leading to a failure to recognize their limitations or errors. This overconfidence can result in poor decision-making, particularly in high-stakes scenarios
Brolly Academy
Conclusion
Generative AI vs Predictive AI represent two distinct yet interconnected areas within artificial intelligence. Generative AI focuses on creating new content and fostering creativity, while predictive AI excels at analyzing historical data to forecast future outcomes. For businesses, understanding the differences between Generative AI vs Predictive AI and how they can complement each other is crucial for effective AI utilization. As advancements continue, both types of AI will play increasingly significant roles across various industries, driving innovation and enhancing decision-making. Combining the strengths of Generative AI and Predictive AI could unlock new possibilities, transforming how we leverage technology and data in our daily lives.